Nosto Cold Start Pale Ale: Our Journey to Uniting Lovers of Data and Beer
To commemorate the launch of our latest beer, Cold Start, we shed some light on our journey to crafting our very first AI-powered brew: from the importance of a well-rounded beer collection to how we gathered the data that fuels our new recipe.
Over the years, the Nosto team has worked round-the-clock to unite the most brilliant minds in ecommerce and transform online personalization. Not only does our team of 100+ industry experts service thousands of leading retailers across the globe, but we also make some damn good beer in the process.
One of our most treasured accomplishments is the beer fridge in our Helsinki headquarters, which is loaded with a combination of locally sourced brews and Nosto’s own curated masterpieces. Our fridge is a must-stop shop for Nostonians near and far and visitors who want to enjoy a well-deserved, post-work beer together, so it’s always stocked with a variety of brands. However, with so many unique preferences to satisfy, creating the dream beer fridge hasn’t always been the easiest, and filling it with certain flavors that went untouched (a downright crime in the eyes of any beer connoisseur) proved wasteful. For us, it was all or nothing: we needed to offer a selection that everyone would love.
Identifying the Challenge
(and an Elementary-but-Brilliant Game Plan)
The plan manifested itself during a brainstorm session with our Product team.
The challenge: How do we improve the quality of our beer and adjust the quantities of orders to remain efficient?
The intended solution: Hiring a brewmaster to help us create our new recipe.
The actual solution: Abandoning the brewmaster idea and turning to what we already do best — data and software.
As a multi-talented group in various fields of software development, our team’s favored solution was to crowdsource feedback from other colleagues in the Helsinki office virtually, via the HappyorNot app. However, we quickly realized that gamifying beer lovers into providing feedback via an app would be too challenging. We needed a way to build a simple proof-of-concept in order to test and validate the issue at hand — so we dusted off our primary school creativity to get the job done.
After a quick trip to the corner hardware, we set up four large bins in our storage area, each labeled with an emoji face.
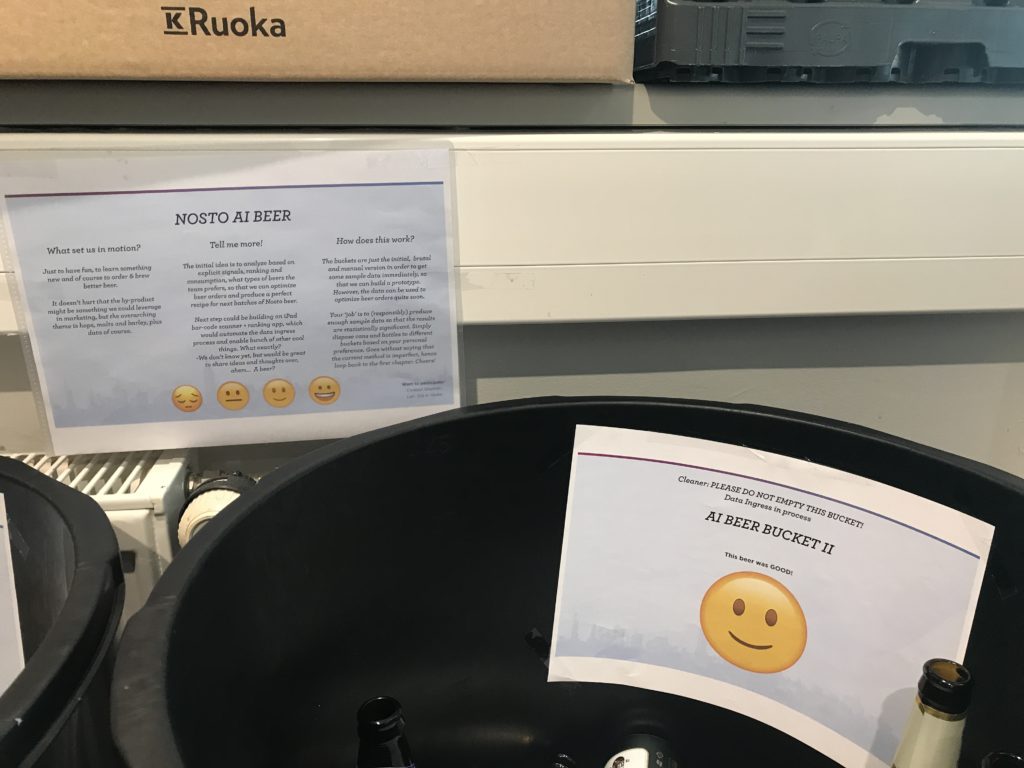
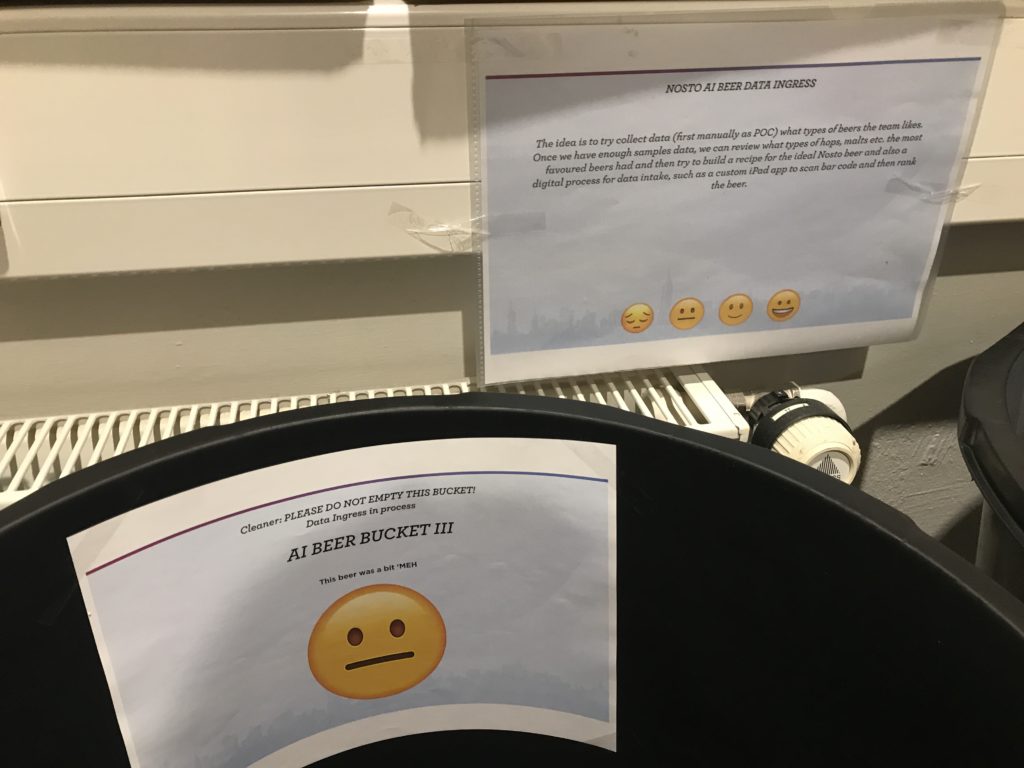
In the Nordics, bottles and cans have a deposit, so our recycling rate is very high. Also, our people generally return used cans and bottles to our storage room in order to keep the office clean. Whenever someone returned a beer bottle, they were expected to place that empty bottle into the bin that best represented their reaction to its flavor (i.e. tasty beer in the ‘happy face’ bin, not-so-tasty beer in the ‘sad face’ bin, as shown above). This made collecting the data simple, inexpensive and quick to deploy.
The process had one surprising and slightly tragic bug: Our cleaning service didn’t get the memo, so they emptied the bins right away. After deploying a much-needed bug fix of chatting with the cleaners about the seriousness of our experiment (and experiencing their amusement over the mission at hand), we were back on schedule! Team members who loved their beers religiously rated their preferred products by categorizing their bottles and cans. After a few weeks, Stephen (our Data Scientist) and I got our hands (quite literally) dirty by counting the bottles and cans, bin by bin, before they were taken away. This produced the first data set for Stephen to play with, and we were one step closer to the brew of our dreams.
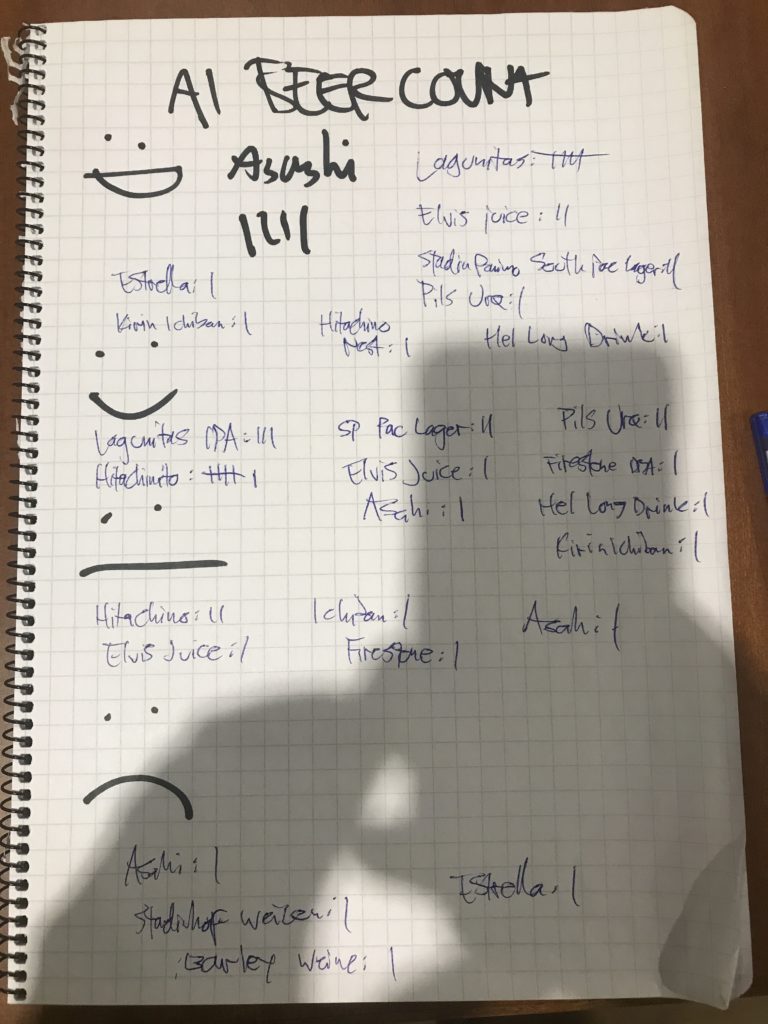
Pre-Beer Brew: Using Our New Data to Test the Right Batch of Flavors
The final step before brewing was to gather a candidate set of beers. “Lucky” for us, Finland has a monopoly on alcohol sales and deliveries for businesses, so we would just need to rely on one inventory regardless. We quickly scraped our local alcohol retailer Alko’s online catalog, which not only contains a long list of beers to choose from, but also the properties of each beer (i.e. hops, malts and bitterness). After that, we were able to score the beers we had previously ordered and then flag beers on the list that were similar to the ones we liked (and different from from beers we disliked). This resulted in a candidate set from Alko’s inventory which depended on the data at-hand.
Since we were using a limited set of beer properties for our scoring, we needed to–like most AI-driven processes–add a human touch to the process. The majority of the shortlisted beers were almost exactly the same as the ones in our top five list, but who really drinks just one type of German pilsner? We clearly needed to add a way to incorporate a bit more variation in the selected order.
Alko doesn’t have an API to allow order automation, so the order process needed to be manual. One could also say that, while computers are smart, they can’t completely replace the human touch– especially when it comes to sensitive topics like beer. While it was quite helpful to get a relevant shortlist, at the end we wanted to curate the final purchase order to have some variation. With that said, we were exposed to some beers that we normally would not have considered, so the relevant shortlist helped the human curator (i.e our team) to be a bit more adventurous with our selections.
Beer Order #1: A Success! (Well, Almost.)
In our first test batch, we did not consider the price of the beer (oops), so purchased some pretty expensive brews. This meant that we bought less beer, which resulted in the beer running out even faster. In subsequent orders, we included price as part of the optimization problem in order to strike a nice balance between price and quantity without hurting the beer selection.
The scores given to the beers improved, and based on our qualitative analysis, a variety of beers were consumed steadily as opposed to the most popular ones running out the moment our beer fridge met its first thirsty visitor. After repeating the process for some months, we had collected a reasonable amount of data which resulted in a solid selection of beers that our team liked. From there, we incorporated those preferences into our beer recipe and started brewing the newest addition to the Nosto beer collection.
‘Cold Start’: Solving a Complex Problem with a Delicious Solution
While our journey to craft a brew loved by many was a challenging one, finding an initial solution proved to be less complicated than we imagined. As our team continues to grow, we will continue to improve our process at a more granular level and use data to influence the future of our treasured brew collection.
Have we cracked the code on brewing a completely AI-powered beer? We’re getting there! We acknowledge that the data set used for our latest beer recipe, and our overall methodology, still has a ways to go. For this reason, we decided to name our new beer Cold Start — to serve as a nerdy-but-still-relevant reminder that this is only the beginning of our journey to use data to transform more than just our personalization solution.
Find yourself in any of Nosto’s 7 home cities? Pop into our office and enjoy our latest brew with us!